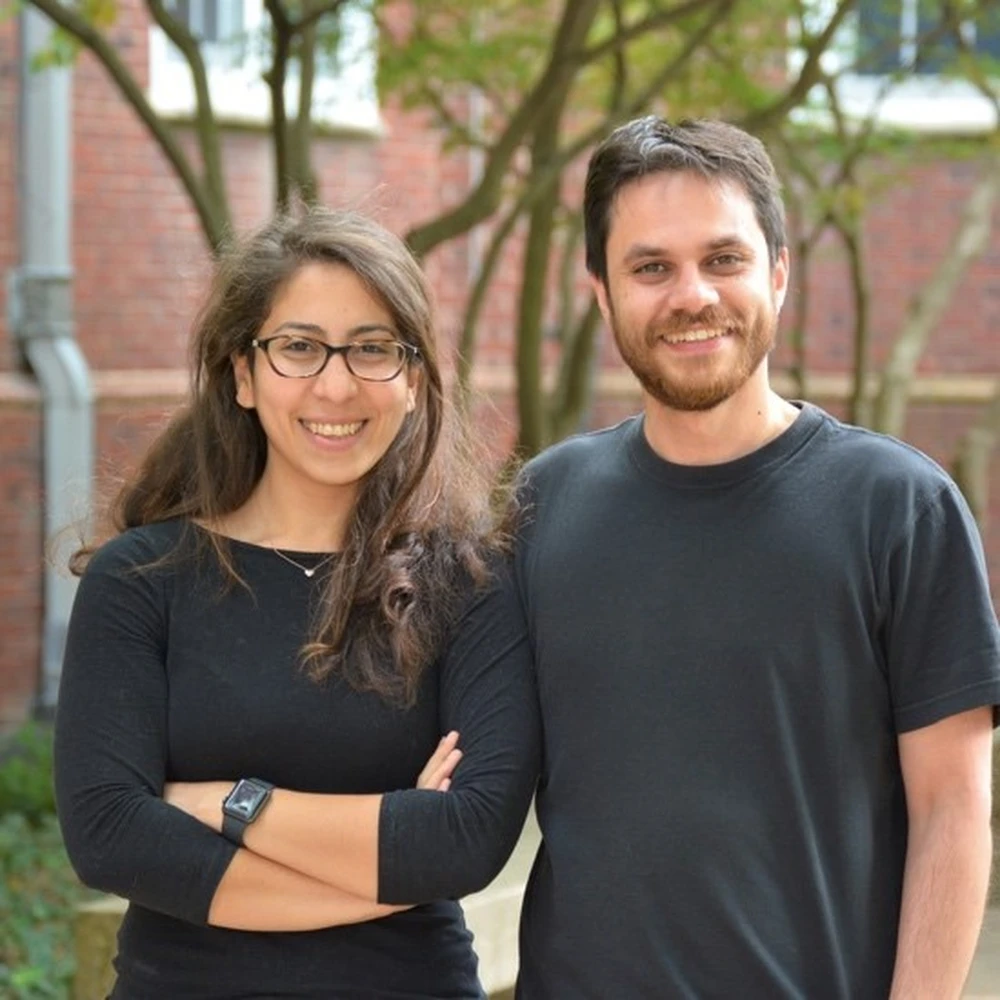
We (Abdollah Farhoodi and Nazanin Khazra) are two PhD students in Economics that have been co-teaching the Applied Machine Learning in Economics course from Spring of 2019 to Spring of 2020. This course teaches students to work with fundamental ML models and to use these models in answering economic questions. The goals of this course are for students to leave with a great knowledge of coding, interpreting and understanding ML models, and this culminates in the completion of a full, publishable research paper where students choose real world datasets to work with as a basis for their investigation.
Throughout the semester, students submit bi-weekly reports that receive comprehensive commentary and feedback from the TAs. Every report is reviewed three times in total, twice by different TAs and once by an instructor, and the students are encouraged to follow up with TAs on a regular basis. By the end of the semester, we hope this process ensures that every student has a polished research paper that begins to answer an important economic question using the skills that they learn in this course. Graduates of this class have been able to leverage their papers to get accepted to prestigious graduate schools and find great jobs.
One of the keys to the success of this course is our amazing TAs. We rely upon them to carry out the duties that we entrust them with, and we have come to expect each TA to act as a capable instructor in their own right. Not only do they enrich this course with their guidance and assistance, they all cooperate, take initiative, and truly go above and beyond to help students learn. We have a team of talented, motivated individuals, and you can read more about each of them below!
Derek
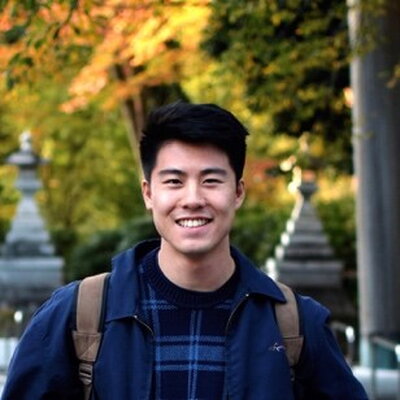
Hi! I’m Derek, a third-year undergraduate student double majoring in Econometrics and Statistics, with a minor in Computer Science. I currently work as the head TA for this course, the econometrics tutor for the Economics Department, and a collaborator at the AbbVie Innovation Center, where I am developing a machine learning web application for drug discovery.
Before taking this course, I would never have imagined myself embedded in this quantitative, data-centered, and technology-oriented community. In fact, I could be considered a luddite among my peers having grown up without video games, a laptop, or even a working family desktop computer. I cared about people, not machines, so I figured my time was better spent reading articles on political economics, evolutionary biology, philosophy, personality, and otherwise staying away from technology at university.
When I decided to take this course, I thought it might make for an interesting elective or, at the very least, a useful subject to broach. Everyone seemed to be singing the praises of machine learning after all. Like most people, I had no understanding of “machine learning” at the time, but as someone accustomed to the data modeling approach taken by classical econometric analysis, I thought of the algorithmic approach of machine learning as a black box—something viewed in terms of its inputs and outputs, without any knowledge of its internal workings.
In taking this course, I realized that I was wrong: Machine learning is nothing more than a class of computational algorithms, and what matters in practice is the way they are implemented. While my initial perception of the subject was accurate (pun intended) in a prediction context, it falls short in a social science context where, much like classical econometrics, the treatment of machine learning places a premium on inference and interpretation. All that is to say, I don’t want anyone who is thinking about taking this course to be discouraged by the idea of machine learning. This course is more similar than one might think to other econometrics courses, and it is kind to students with limited programming experience, like me at the time!
What I loved most about this course is that it provides an opportunity for students to pursue original, independent research for the semester and, perhaps, beyond. I personally took this as motivation to string together a paper exploring the potential of linking personalized healthcare to the opioid crisis, but I think that the process offers an invaluable learning experience regardless of the topic. Oddly enough, my favorite parts of being a TA are learning about what students choose to investigate and then encouraging independence and enthusiasm in their learning.
Ultimately, my experiences in this course have imparted a sense of how powerful, insightful, and versatile technology can be depending on the way you apply it, and in turn, this has helped me understand that if I care about people, then I might also care about machines… because wielding machines intelligently can allow people do a much better job of helping others.
Ashna
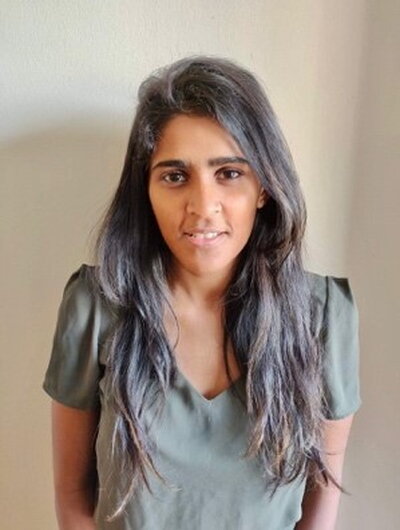
My name is Ashna Anil, and I am a senior double majoring in Economics and Mathematics and a minoring in Computer Science. I took Applied Machine Learning in Economics in Fall 2019, loved the class, and hence became a TA this semester. From this experience, I have not only enhanced my leadership and teaching skills, but I have also been exposed to a plethora of research topics. As a student, I chose a topic I was interested in and had the chance to explore this topic at different depths, practice cleaning data, run different models, and formulate a professional research paper at the end. This definitely boosted my interest in research work.
I was able to pursue this interest further as a TA by mentoring students to do the same with topics that I have not explored before. This opportunity allowed me to dig deep into new areas of research, understand the thinking process of other students, see how the same models would work on different data, and comprehend the economic implications for the different research questions. I was faced with unique difficulties and errors and was able to understand and overcome this, yet again boosting my self-confidence and my interest in applied work and understanding economic problems.
Celia
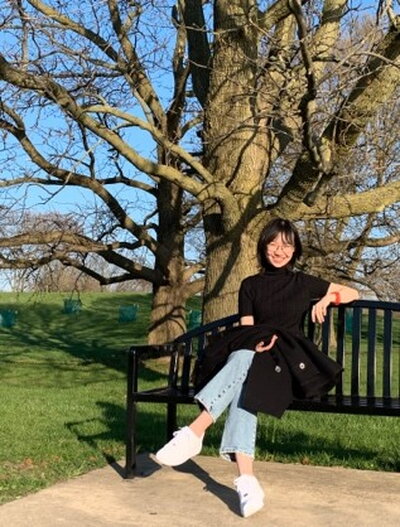
I’m Celia, a junior, major in Econometrics and Quantitative Economics. Being a TA for AML is a magical journey for me. It’s a journey of patience. Getting a good prediction result is never an easy thing. Data cleaning is a struggle, yet fun. The process of tuning parameters and searching for the best models, from shrinkage to tree-based methods to neural net, unveiled its charm gradually. It’s a journey of self-improvement. The challenge of debugging and giving specific suggestions based on different students’ topics enhances my problem-solving skill by solidifying my knowledge of each model.
It’s also a journey with gratitude. I always found the attitude and effort that students put into their work inspiring. I also appreciate the trust given by our instructors as well as mentors, Nazanin and Abdollah, in providing us with the opportunity to see the world on the other side of the table. If you’re thinking about the world as a puzzle, then machine learning is a secret weapon to solve it. The idea fascinates me enough to want to keep digging into this field and solving more puzzles in this world as a data scientist.
Daniel
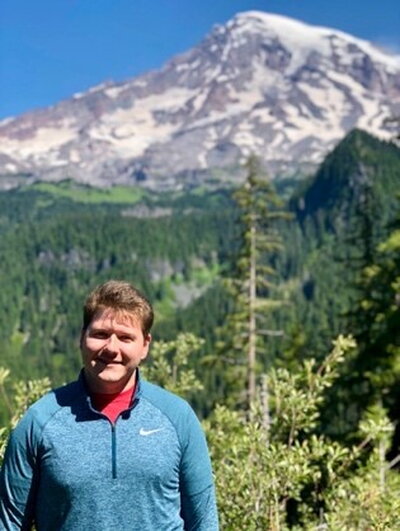
Hi all, I’m Daniel Stumpf, a senior here at UIUC studying Econometrics and Quantitative Economics with a minor in Computer Science. I transferred into the department of Economics as a sophomore with a passion for problem solving. I had the opportunity to have a prevalent role in Econ Ambassadors, be part of a large research team, and work with two courses as an undergraduate TA. Most recently I have been a TA for Econ 490 AML, and I have thoroughly enjoyed delving deeper into some of the topics covered in the course and exploring different applications of the learned models.
This course, as a student and a TA, has changed my perspective on modeling and writing. As a student, I developed a full research paper covering different modeling techniques from simple regression to neural networks, and as a TA I have improved these skills by guiding students in their process. These research papers cover 18+ topics, spanning police brutality ratings, blockchain movement prices, bitcoin, medical insurance expenditures, sports statistics, and more. Reinforcing topics with students, walking through and debugging code, and revising and commenting papers have all encouraged me to find more situations and applications of machine learning techniques. It has been a great experience working with the other TA’s and the Instructors and seeing the student’s growth throughout the semester.
Jia
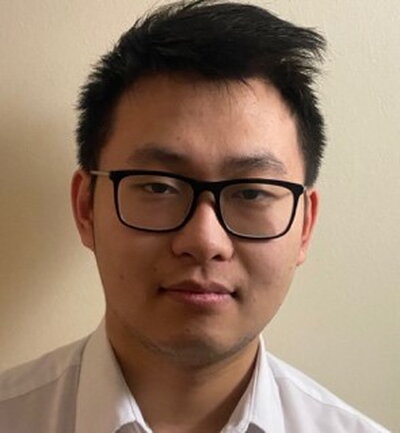
Hi, I'm Jia Li, a senior double majoring in Statistics and Economics. I took Applied Machine Learning in Fall 2019 and became a Teaching Assistant for the course in Spring 2020. Although I had prior experience programming in R, this class improved my coding skills and taught me how to apply different machine methods on large data sets.
Aside from all this, the most important thing I learned was how to analyze data and provide meaning to numbers. Exploring a real data set from data preprocessing to statistical modeling has boosted my confidence in doing research and my interest in machine learning. In fact, my experience throughout the course has taught me the skills needed to explore various data sets for applied research on my free time.
As a teaching assistant, I held weekly in-person office hours offering any guidance students needed. Whether it was helping them debug their code or even better understanding the difference between certain machine learning techniques, it was a pleasure being that helping hand students needed to get through the course and their research project. The most rewarding part was seeing students start the course confused to gradually understanding the various methods and applying them. Learning how to teach, work with, and understand other people's thought process also developed the soft skills that are often overlooked. It has been a wonderful and transforming experience.
Lexi
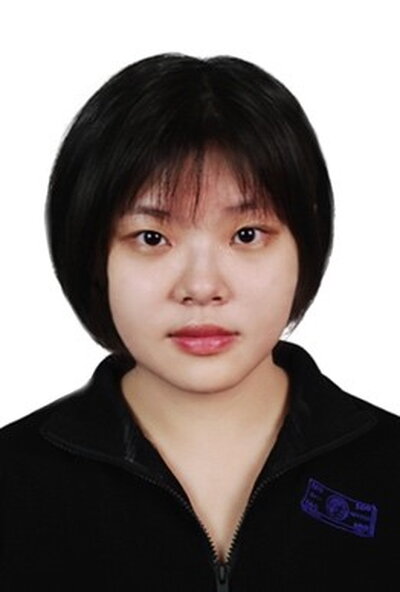
Hi, my name is Lexi, and I am a senior in Statistics and Economics. I took Applied Machine Learning (AML) last semester, and currently, I am serving as a teaching assistant for the class. Through AML, Nazanin and Abdollah explain how powerful the tools of statistical learning could be, and the appeal is mesmerizing. From cleaning data to modeling to forming a paper, the process builds my confidence in research and motivates me to take initiative outside of school to approach applied works. I have enjoyed my experience both taking and assisting the class, as it greatly shapes my academic preference, and it is fun.
Xinyi
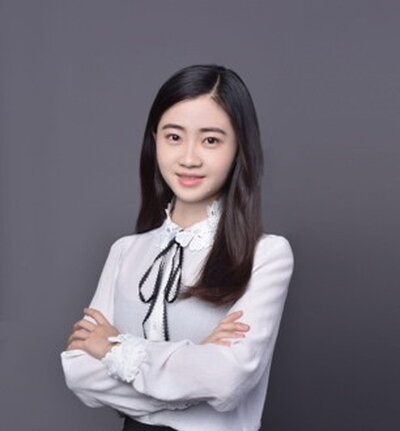
I'm Xinyi Li, a senior double majoring in Mathematics and Economics. I took Applied Machine Learning last semester, where I had the opportunity to gain a deeper understanding of Ethereum Blockchain using Machine Learning methods. As a Teaching Assistant, I also enjoyed learning about various datasets and helping students with their codes.
After taking this course, I have applied models, such as Support Vector Machine, XGBoost, and Neural Network, to predict financial distress in my quantitative analyst internship. Moreover, this research experience gives me a solid background for my future study in Financial Mathematics at the University of Chicago, where I will be confident in learning more Machine Learning algorithms.